Book review: We, the Robots? Regulating Artificial Intelligence and the Limits of the Law, by Simon Chesterman (Cambridge University Press, 2021)
From Tesla’s self-driving cars that can comfort your dog, to OpenAI’s large language model that writes decent essays and code, more and more Artificial Intelligence products are pushing the limit of what machines can do in our life. Meanwhile, powerful new AI tools are being incorporated into routine aspects of government administration, law enforcement, and legal practice to save human time and effort, improve operational efficiency, and save costs.
These exciting developments, however, come with various degrees of risk and uncertainty. Already, we have seen road accident injuries for which no “persons” could be legally held at fault, wrongful criminal arrests, unlawful government debt notices that devastated livelihoods for years to come, and a stock market crashing so quickly that $1 trillion market value was lost in minutes.
The recent book of Simon Chesterman, We, the Robots?, is a forceful response to these new issues. It offers a multi-faceted, concrete, and practical guide to thinking about how to make our socio-legal systems “AI-ready” using mostly the regulatory tools we already have. The resulting book is a wealth of rigorously researched, well-explained regulatory insights into how to restore public control over a range of AI technologies.
A useful contribution of the book is a “trichotomous” categorisation of AI challenges centred around practicality, morality and legitimacy, which can inform the basis for three types of regulatory strategies.
Only an informed user can see the output of an AI system not as a decision, but as information to engage, interrogate, and instrumentalise in their own decision-making process.
Firstly, practicality guides strategies towards many task-specific AI technologies with high utility but relatively straightforward mandates, such as to protect human safety and increase efficiency. An example is self-driving cars. For such AI technologies, redesigning the entire foundation of law to accommodate AI entities could be less practical than adapting existing regulatory tools, such product liability law and mandatory insurance scheme.
But regulating AI is not just about risk management. Sometimes, moral “red lines” need to be drawn to constrain certain developments. In such AI technologies, the moral risks are so complex, and the stakes are so high that creative risk management would not suffice. In the case of lethal autonomous weapons, for example, fundamental human rights and dignity are at stake. Even if AI weapons can perfectly comply with the law of war, should we ever “outsource” life-and-death decisions to machines? For moral lines to be drawn on high-risk AI, Chesterman calls for international actions, coordinated via a global institution.
But what of all the AI technologies that fall in between? For many AI applications, the moral risks are not as high as autonomous weapons, while the public interests to defend are not as straightforward as self-driving cars. Here, the temptation to abrogate responsibility to machines is often particularly strong, while the harms it causes to the public is not always clear.
Algorithmic risk assessment tools used in bail decisions and credit rating is a prominent example. With the appearance of neutrality, objectivity and accuracy, such technologies can claim authority simply by virtue of being advanced statistical tools. But therein also lies their limits – that they are, at least in the foreseeable future, no more than advanced statistical tools. It is now a truism that algorithmic decisions are only as good as their underlying training data. If the training data mirrors real-world systemic biases, so will the outcome of the algorithmic system.
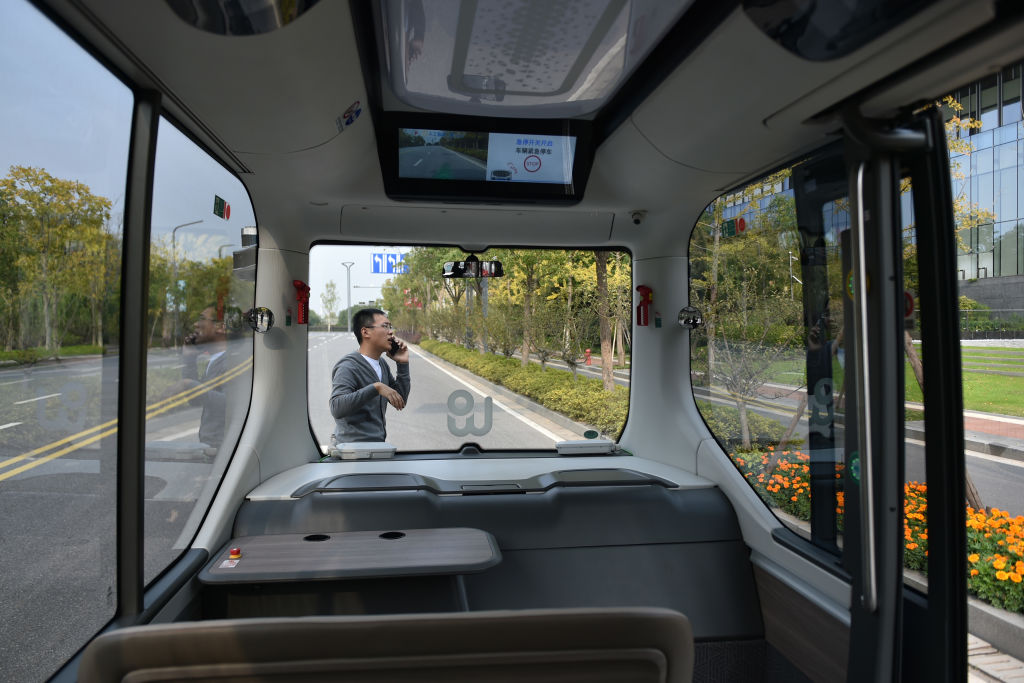
To this class of problems, Chesterman stresses the importance of process legitimacy. In contexts where public interests are affected, legitimacy of decision-making “lies in the process itself”, where a capable human being can stand behind the exercise of discretion and be held accountable. Here, regulations must ensure process transparency and human control.
“Process legitimacy” is perhaps the most original idea from Chesterman’s trichotomy, as it puts in sharp relief the value of our own reasoning, discretion and deliberation. While human decision-making is often just as fallible as AI’s, Chesterman notes that there is something fundamentally different about human reasoning compared to AI, even those capable of deep learning and complex information processing.
An algorithmic risk assessment tool distils patterns from past human decisions and their outcomes to inform future decisions. A human judge, however, not only examines past information, but also exercise discretion by weighing uncertain values in complex social environment. Here, as Chesterman asserts, relying on AI reduces legal reasoning to “a kind of history” rather than the “forward-looking social project” that it is.
Chesterman’s conclusion may induce many to ask if AI could ever be a part of forward-looking human projects without confining us to the shadows of our past. The answer, in my opinion, is an emphatic yes. Advanced statistical models are powerful informational tools that empower us to make better decisions, but only if we truly understand our tools.
Herein lies a current blind spot – and future extension – of current thinking on AI regulation. This missing aspect is social policy, an integral part of which is public education. It is indeed important to discuss how regulations can protect the public from AI, but the flipside of that is also crucial: how regulations can prepare the public to rise to the challenge of AI.
As AI tools inevitably become more sophisticated and permeate more spheres of life, only an informed user can see the output of an AI system not as a decision, but as information to engage, interrogate, and instrumentalise in their own decision-making process. This entails a critical attitude towards AI and some internal understanding of how it is designed and trained, as well as how it is likely to fail and display biases. We will need more and more such “informed users”, staffed in various “traditional” roles that are becoming AI-assisted or partially automated. In other words, we need to increase the basic threshold of technical literacy and education, so that the “average” computer users of the near future are also true masters of their “AI minds”.
Hence, the continuance of human agency and authority lies not only in external protection, but also in our internal knowledge and skills, which social policy and regulation should support and foster.
Overall, We, the Robots? is a thought-provoking account of how governments can safeguard public interests and maintain legitimacy in the age of AI. While it does not provide groundbreakingly novel insights, it is a comprehensive and engaging read for anyone interested in better understanding AI, its impact on our legal landscape, and some of the thorniest new issues confronting regulators today.